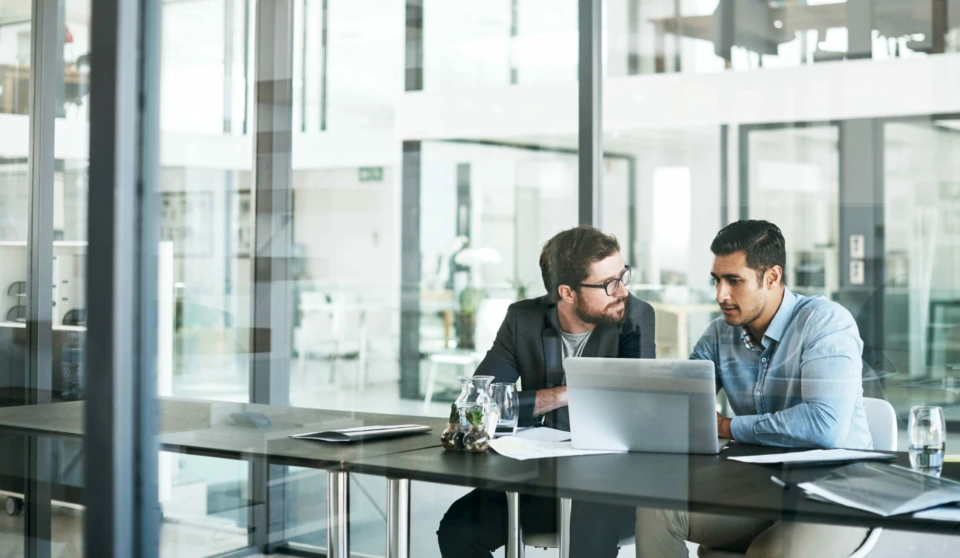
Insights
Access the market perspectives and expert knowledge that can help you stay ahead.
-
Subscribe for more insights
Get the latest insights from Chatham delivered to your inbox weekly.
1-24 of 182
results
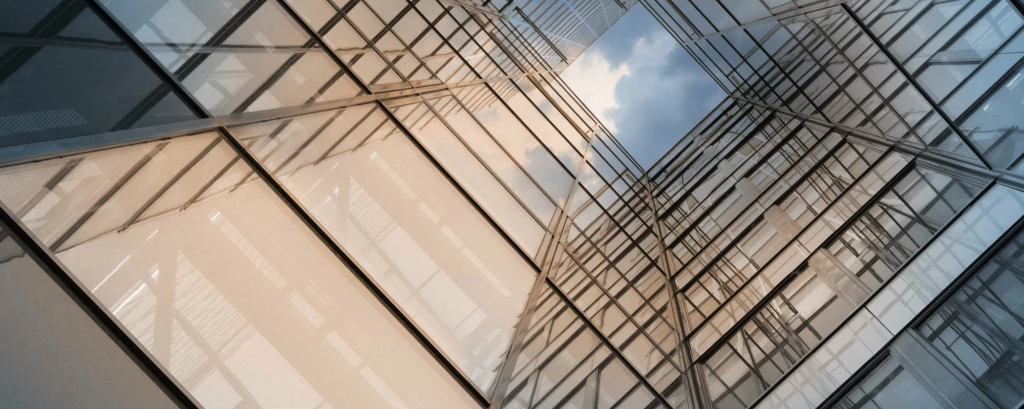
What we do
Discover how we effectively manage and mitigate financial risk with advisory, process, and technology.
Our solutions
Discover how Chatham’s solutions can transform our knowledge and expertise into your opportunity.